title: “Benchmarking Iteration” |
output: rmarkdown::html_vignette |
self_contained: yes |
vignette: > |
% |
% |
% |
This document illustrates the differences in performance between
iterors
and previous packages
iterators
/itertools
/itertools2
.
Basic gains come from the nextOr
method avoiding the need
for tryCatch
, as well as the fact that iterors can be used
simply as functions, skipping S3 dispatch. Additionally, over the
process of curating the iterors
package, several iterator
implementations have been optimized for performance.
Because this is a more computationally intensive vignette, it is
built manually and included in the released package statically. The
Rmd
source is in the Git repo under the
scratch
directory; knit it manually and copy it into the
vignettes directory to publish. When this document was last built, it
was under these conditions:
- iterors version 1.0, git revision 257dc955 ()
- itertools2 version 0.1.1
- itertools version 0.1.3
- OS: Linux 5.4.0-148-generic
- CPU: Intel(R) Core(TM) i5 CPU M 520 @ 2.40GHz.
- R version 4.3.0 (2023-04-21), svn rev 84292
Basic iterators (self-contained/memory-backed)
Consuming iterators
Since the following tests will use different versions of
icount
, iter_consume
, and
as.list
, first check how close those functions are.
as.list.bench <- microbenchmark::microbenchmark(
iter_consume = iter_consume(iterators::icount(3000)),
iterors.consume = iterors::consume(iterors::icount(3000)),
iterators.as_list = as.list(iterators::icount(3000)),
iterors.as_list = as.list(iterors::icount(3000)),
times = 10)
plotit(as.list.bench)
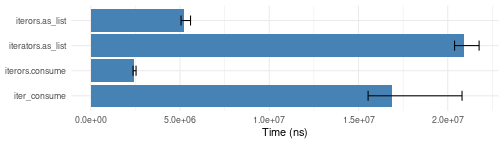
plot of chunk unnamed-chunk-2
Note that we are using our own function iter_consume
, as
itertools::consume
has poor performance due to invoking
try
on every item.
Simple iteration over a vector
vector.bench <- microbenchmark::microbenchmark(
iterators = iter_consume(iterators::iter(1:1000)),
iterors = iterors::consume(iterors::iteror(1:1000)),
times = 10)
plotit(vector.bench)
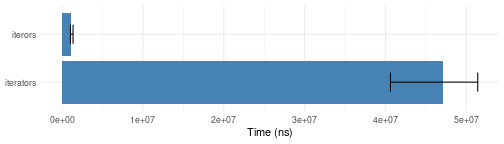
plot of chunk unnamed-chunk-3
Chunking a vector
chunk.bench <- microbenchmark::microbenchmark(
itertools = iter_consume(itertools::isplitVector(1:6000, chunkSize=17)),
iterors = iterors::consume(iterors::iteror(1:6000, chunkSize=17)),
times = 10)
plotit(chunk.bench)
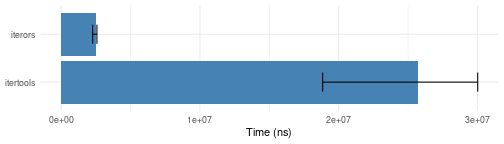
plot of chunk unnamed-chunk-4
Extracting rows or columns
arr <- array(1:1000000, c(1000,1000))
slice.bench <- microbenchmark::microbenchmark(
iterators.rows = iter_consume(iterators::iter(arr, by="row")),
iterators.cols = iter_consume(iterators::iter(arr, by="column")),
itertools.array_rows = iter_consume(itertools::iarray(arr, MARGIN = 1)),
itertools.array_cols = iter_consume(itertools::iarray(arr,MARGIN = 2)),
iterors.rows = iterors::consume(iterors::iteror(arr, by=1)),
iterors.cols = iterors::consume(iterors::iteror(arr, by=2)),
times = 10)
plotit(slice.bench)
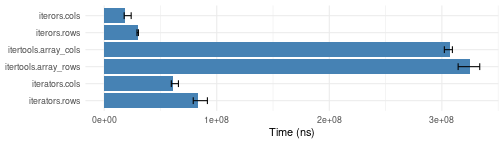
plot of chunk unnamed-chunk-5
Split matrix into chunks
arr <- array(1:1000000, c(1000,1000))
array_chunks.bench <- microbenchmark::microbenchmark(
itertools.rows = iter_consume(itertools::isplitRows(arr, chunkSize=7)),
itertools.cols = iter_consume(itertools::isplitCols(arr, chunkSize=7)),
iterors.rows = iterors::consume(iterors::iteror(arr, by=1, chunkSize=7)),
iterors.cols = iterors::consume(iterors::iteror(arr, by=2, chunkSize=7)),
times = 10)
plotit(array_chunks.bench)
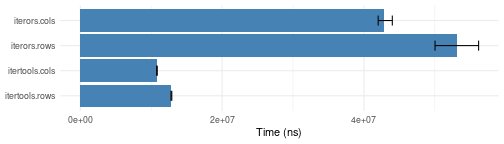
plot of chunk unnamed-chunk-6
Enumerating N-D array indices:
icountn.bench <- microbenchmark::microbenchmark(
iterators = iter_consume(iterators::icountn(c(4,5,6,7))),
iterors = iterors::consume(iterors::icountn(c(4,5,6,7))),
times =10)
plotit(icountn.bench)
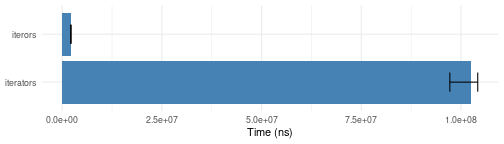
plot of chunk unnamed-chunk-7
Cartesian product of vectors
igrid.bench <- microbenchmark::microbenchmark(
# itertools2 = iter_consume(itertools2::iproduct(1:10, letters, month.name)),
itertools = iter_consume(itertools::product(1:10, letters, month.name)),
itertools2 = iter_consume(itertools2::iproduct(1:10, letters, month.name)),
iterors = iterors::consume(iterors::igrid(1:10, letters, month.name)),
times = 10)
plotit(igrid.bench)
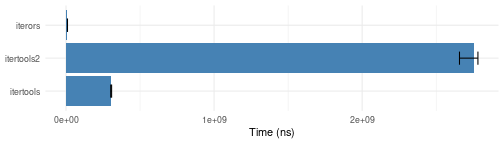
plot of chunk unnamed-chunk-8
Random number generation
When independent=TRUE
there is a slight amount of extra
work in saving and restoring the random number generator settings. The
difference becomes smaller the larger “n” you pick.
sampling.bench <- microbenchmark::microbenchmark(
iterators = iter_consume(iterators::irunif(100, count=100)),
iterors.independent = as.list(iterors::irunif(100, count=100, independent=TRUE)),
iterors.dependent = as.list(iterors::irunif(100, count=100, independent=FALSE)),
times = 100)
plotit(sampling.bench)
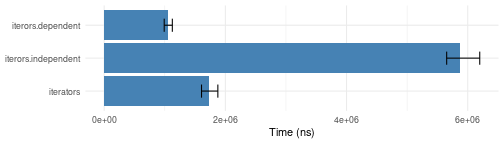
plot of chunk unnamed-chunk-9
What are the costs of speed here? here?
library(profvis)
Higher order iterators / iterator functions
Chaining iterators end-to-end
chain.bench <- microbenchmark::microbenchmark(
itertools = iter_consume(
do.call(itertools::chain,
lapply(1:50, iterators::icount))),
itertools2 = iter_consume(
do.call(itertools2::ichain,
lapply(1:50, iterators::icount))),
iterors =
iterors::consume(do.call(iterors::i_chain, lapply(1:50, iterors::icount))),
iterors.collapse =
iterors::consume(iterors::i_concat(iterors::i_apply(1:50, iterors::icount))),
times=10)
plotit(chain.bench)
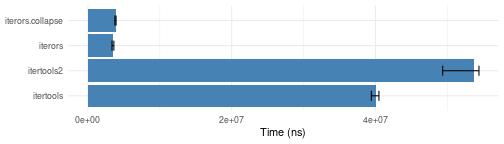
plot of chunk unnamed-chunk-10
Keeping or dropping items according to a criterion
i_keep.bench <- microbenchmark::microbenchmark(
iterators = as.list(itertools::ifilter(\(x) floor(sqrt(x))^2 == x, iterators::icount(5000))),
iterors = as.list(iterors::i_keep(iterors::icount(5000), \(x) floor(sqrt(x))^2 == x)),
times = 10)
plotit(i_keep.bench)
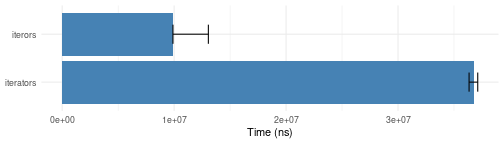
plot of chunk unnamed-chunk-11
Select only unique values
iterors::i_unique
uses a hash table for better
performance.
i_unique.bench <- microbenchmark::microbenchmark(
iterators = {
it <- itertools2::iunique(iterators::isample(1:100, 1))
as.numeric(itertools2::take(it, 100))
},
iterors = {
it <- iterors::i_unique(iterors::isample(1:100, 1, independent=FALSE))
iterors::take(it, 100, "numeric")
},
times=10)
plotit(i_unique.bench)
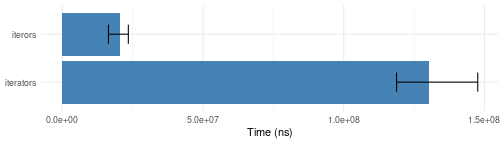
plot of chunk unnamed-chunk-12
i_recycle
Note that itertools2::icycle
only works for
self-contained vector-based iterators, while the other two use a general
purpose buffer.
icycle.bench <- microbenchmark::microbenchmark(
itertools2 = as.list(itertools2::icycle(iterators::icount(20), times = 20)),
itertools = iter_consume(itertools::recycle(iterators::icount(20), times = 20)),
iterors = iterors::consume(iterors::i_recycle(iterors::icount(20), times = 20)),
times = 20)
plotit(icycle.bench)
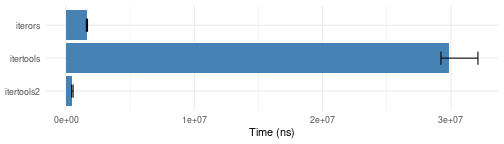
plot of chunk unnamed-chunk-13
i_tee
Note that itertools2::tee
only works for vector-based
iterators it knows how to clone, while iterors::i_tee
uses
a general purpose buffering mechanism.
i_tee.bench <- microbenchmark::microbenchmark(
itertools2={
iter_consume(
do.call(itertools::chain,
itertools2::itee(1:20, n=20)))
},
iterors={
iterors::consume(
iterors::i_concat(
iterors::i_tee(iterors::icount(20), n=20)))
},
times=20)
plotit(i_tee.bench)
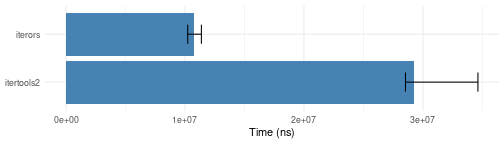
plot of chunk unnamed-chunk-14
Sliding window iterators
iterors
uses specialized routines for n=2 and n=3. For
n=4 and above, a general purpose queue is used.
marks=c(alist(
itertools2.w2={iter_consume(itertools2::ipairwise(1:200))}),
if (exists("itripletwise", asNamespace("itertools2"), inherits=FALSE)) {
alist(itertools2.w3={iter_consume(itertools2::itripletwise(1:200))})
},
alist(
iterors.w2={iterors::consume(iterors::i_window(1:200, 2))},
iterors.w3={iterors::consume(iterors::i_window(1:200, 3))},
iterors.w4={iterors::consume(iterors::i_window(1:200, 4))},
iterors.w50={iterors::consume(iterors::i_window(1:200, 50))}))
i_window.bench <- microbenchmark::microbenchmark(list=marks, times=10)
plotit(i_window.bench)
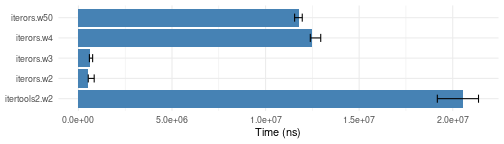
plot of chunk unnamed-chunk-15